Addressing Bias and Fairness in AI: Ethical Considerations
Artificial Intelligence Ethics
As someone deeply intrigued by the ever-evolving world of technology, I find myself captivated by the intricate dance between ‘Bias and Fairness in AI. In an era characterized by the burgeoning influence of artificial intelligence (AI), ethical considerations loom large on the horizon. As AI continues to permeate every facet of our lives, bias and Fairness have taken centre stage in the discourse surrounding this transformative technology. Ethical Considerations in AI: Bias and Fairness is a crucial examination of the ethical dimensions within the realm of AI, aiming to shed light on the complex interplay between algorithms, data, and human values.
With its incredible power to process vast amounts of data and make decisions, AI is not immune to inheriting and perpetuating societal biases in the data it’s trained on. This introduction sets the stage for a deep dive into the multifaceted world of AI bias, encompassing algorithmic, data, and user-related biases. We’ll explore real-world examples, the profound consequences of biased AI systems, and the critical role of data in perpetuating or mitigating bias. Join us on this enlightening journey as we delve into the ethical intricacies of AI, uncover the hidden biases that shape its outcomes, and discover the strategies and solutions for a more equitable and just AI-driven future.
From Code to Conscience: Ethical AI and Fairness
1. Understanding AI Bias:
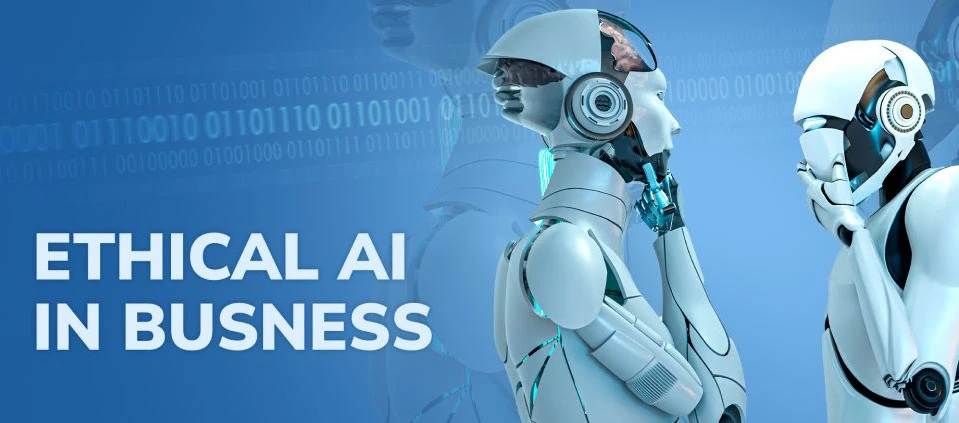
Understanding AI bias is pivotal in grasping the ethical complexities of artificial intelligence. AI bias refers to the systematic and unfair favouritism or discrimination AI algorithms exhibit in their decision-making processes. This bias can manifest in various forms, including racial, gender, socioeconomic, or other forms of discrimination, often reflecting historical biases in the data used to train these algorithms. The origins of AI bias are deeply rooted in the data fed into AI systems. Biased training data can reinforce and perpetuate societal prejudices, leading to skewed and unfair outcomes.
It’s imperative to recognize that AI systems aren’t inherently biased; instead, they learn patterns and associations from the data they’re exposed to. Understanding AI bias involves acknowledging these issues, scrutinizing data sources, and employing techniques such as data preprocessing and fairness-aware algorithms to mitigate bias. By unravelling the intricacies of AI bias, we can work towards developing more equitable and just AI systems, fostering a future where technology upholds ethical standards and promotes inclusivity.
2. The Role of Data in Bias:
The role of data in bias is fundamental to understanding how biases can permeate and manifest in artificial intelligence (AI) systems. Data serves as the lifeblood of AI, influencing the algorithms’ behaviour and outcomes. When data used for AI training contains biases, these biases can be learned and replicated by the AI, leading to biased decision-making.
Data can introduce bias in multiple ways:
- It may reflect historical or societal biases in the real world, encoding prejudices into the AI’s understanding.
- Data collection methods can inadvertently introduce bias, such as underrepresenting certain demographics or perspectives.
- Data preprocessing choices, like data cleaning or feature selection, can inadvertently amplify or diminish existing biases.
Recognizing the role of data in bias is crucial for developers and researchers. It calls for rigorous data collection, transparency in sourcing, and proactive measures to de-bias datasets. AI systems can only be designed to minimize biases, promote Fairness, and contribute positively to society by addressing these data-related challenges.
3. Algorithmic Fairness:
Algorithmic Fairness is a critical concept in artificial intelligence and machine learning, emphasizing the need to ensure equitable and unbiased outcomes in AI-driven decision-making processes. It involves designing AI algorithms and models to avoid discrimination, prejudice, or favouritism towards any specific group or individual.
Key aspects of algorithmic Fairness include:
- Fairness Metrics: These are quantitative measures used to assess the Fairness of AI algorithms. Common metrics include disparate impact, equal opportunity, and demographic parity. These metrics help evaluate whether AI systems treat different groups of people fairly.
- Bias Mitigation: Algorithms are designed to reduce or eliminate bias in predictions or decisions. Techniques like re-weighting, re-sampling, and adversarial training can be employed to make algorithms more equitable.
- Trade-offs: Achieving perfect Fairness may come at the cost of other important factors like accuracy. Algorithmic Fairness often involves striking a balance between Fairness and performance, as overly aggressive fairness measures can decrease overall system accuracy.
- Fairness Considerations: Developers must be mindful of the potential sources of bias in data, feature selection, and algorithm design. Ethical guidelines and best practices should be followed throughout the development process.
Algorithmic Fairness addresses historical and societal biases that may be ingrained in the data used for AI training. AI systems can better serve diverse populations and contribute to a more equitable future by prioritizing Fairness.
4. The Human Element: Designing Ethical AI
“The Human Element: Designing Ethical AI” underscores the indispensable role of human judgment and ethical considerations in developing artificial intelligence (AI) systems. While algorithms and data drive AI technologies, humans shape their ethical framework, guiding their behaviour and ensuring responsible use. Designing Ethical AI begins with the individuals, teams, and organizations involved in AI development. Ethical guidelines and principles must be integrated into the very fabric of AI projects, encompassing transparency, accountability, and Fairness. Diverse and inclusive teams play a pivotal role, as they bring varied perspectives and experiences that can help identify and mitigate bias.
Moreover, the human element encompasses ongoing ethical considerations throughout the AI development lifecycle. It entails regular auditing, monitoring, and evaluation of AI systems to detect and rectify ethical issues that may arise post-deployment. Ultimately, “The Human Element” signifies that AI is a tool wielded by humans, and its ethical implications are a collective responsibility. By recognizing the vital human dimension in AI design and operation, we can foster AI systems that align with our moral and societal values.
5. Mitigating Bias and Ensuring Fairness:
Mitigating bias and ensuring Fairness are central to developing artificial intelligence (AI) systems. These goals aim to address and rectify the potential biases in AI algorithms and decision-making processes, ensuring equitable treatment of all individuals and groups.
- Bias Mitigation Techniques: Mitigating bias involves implementing strategies to reduce or eliminate biases in AI systems. This can include techniques like re-weighting data, re-sampling data, or modifying algorithms to be more sensitive to fairness concerns. These approaches aim to correct imbalances in how the AI treats different groups.
- Debiasing Algorithms: Developers may use specialized debiasing algorithms to preprocess data or modify machine learning models to reduce bias. These algorithms seek to counteract the learned biases by making predictions or decisions that are more equitable.
- Auditing and Transparency: Regular auditing and transparency are essential to bias mitigation and Fairness. It involves continuously monitoring AI systems for bias, documenting the decision-making process, and making the results of these audits accessible to stakeholders and the public.
- Trade-offs: Achieving perfect Fairness can be challenging and may involve trade-offs with other important factors such as accuracy. Striking the right balance between Fairness and performance is critical in bias mitigation efforts.
- Ethical Responsibility: Developers and organizations are responsible for identifying and addressing bias in AI systems actively. Ethical guidelines and best practices should guide the entire AI development process, from data collection to model deployment.
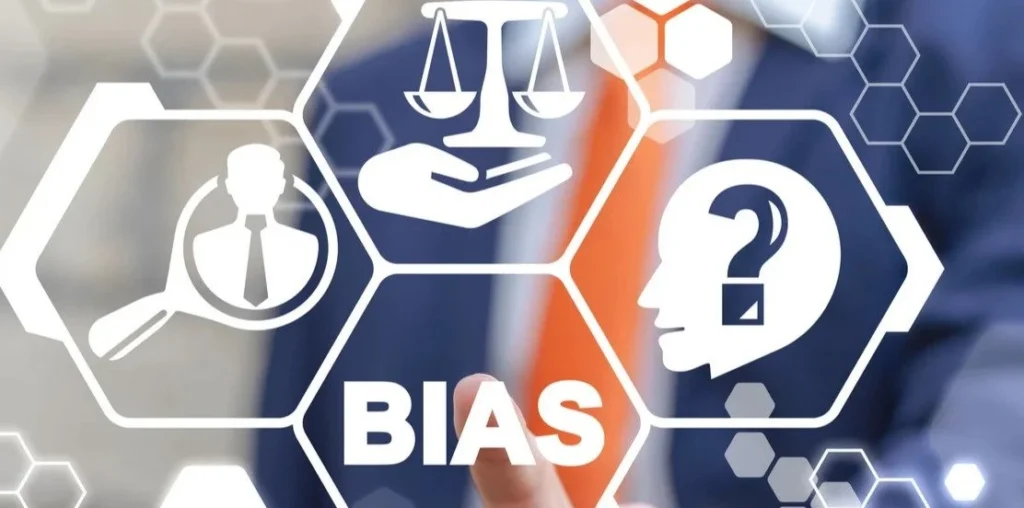
Mitigating bias and ensuring Fairness in AI is an ongoing and evolving process. It requires vigilance, transparency, and a commitment to creating AI systems that promote equity and inclusivity while delivering accurate and valuable results.
6. Ethical AI in Practice:
“Ethical AI in Practice” refers to implementing ethical principles and guidelines in developing, deploying, and using artificial intelligence (AI) systems. It signifies translating ethical considerations into real-world applications to ensure that AI technologies adhere to ethical standards and promote positive societal impacts.
Key aspects of ethical AI in practice include:
- Ethical Guidelines: Following established ethical guidelines and principles throughout the AI development lifecycle. This includes considering Fairness, transparency, accountability, and privacy core values.
- Diverse and Inclusive Teams: Forming diverse and inclusive teams of developers, data scientists, and stakeholders to bring various perspectives and experiences into the AI development process. This helps in identifying and addressing potential biases and ethical issues.
- Bias Mitigation: Implementing strategies to mitigate bias and ensure Fairness in AI algorithms, data, and decision-making processes. Regularly auditing and refining AI systems to reduce unintended consequences is a part of this practice.
- User-Centric Design: Designing AI systems with the end-users in mind, prioritizing user privacy, consent, and user-friendly interfaces that empower individuals to understand and control AI-driven decisions.
- Ethical Use Cases: Selecting and deploying AI in applications that align with ethical values and societal needs and avoiding uses that may result in harm or discrimination.
- Continuous Learning and Improvement: Embracing a continuous learning and improvement culture where AI practitioners and organizations stay up-to-date with evolving ethical considerations and adapt their practices accordingly.
Ethical AI, in practice, aims to bridge the gap between theoretical ethical principles and the real-world impact of AI technologies. By integrating ethical considerations into practical AI development and usage, we can harness the potential of AI for the benefit of society while minimizing harm and bias.
Conclusion:
In conclusion, exploring “Ethical Considerations in AI: Bias and Fairness” has unveiled the complex interplay between artificial intelligence and the ethical imperative of Fairness. In an age where AI exerts an ever-expanding influence over our lives, it is paramount to address the profound challenges of bias and Fairness to ensure that these technologies serve humanity’s best interests. We have delved into the multifaceted dimensions of AI bias, understanding that it can manifest in algorithms, data, and user interactions.
We’ve witnessed how biased AI systems can perpetuate societal injustices and exacerbate discrimination. Furthermore, we’ve explored the pivotal role of data in propagating or mitigating bias, emphasizing the need for rigorous data sourcing and preprocessing. Algorithmic Fairness has emerged as a crucial focus, emphasizing the delicate balance between accuracy and Fairness and the importance of bias mitigation techniques. We’ve also recognized the indispensable human element in designing ethical AI, from diverse teams to ethical guidelines that shape technology’s moral compass.
FAQs:
1. What is AI bias, and why is it a concern?
AI bias refers to the unfair favouritism or discrimination AI algorithms exhibit in their decision-making processes. It’s a concern because it can lead to biased and unfair outcomes, perpetuating societal inequalities.
2. How does bias enter AI systems?
Bias can enter AI systems through biased training data, data collection methods, and algorithm design choices. It often reflects historical or societal prejudices present in the data.
3. What are some real-world examples of AI bias?
Real-world examples include:
- Biased facial recognition systems that misidentify certain racial groups.
- Biased hiring algorithms favouring one gender.
- Biased predictive policing tools that unfairly target specific communities
4. What is algorithmic Fairness, and why is it important?
Algorithmic Fairness is the goal of designing AI algorithms to avoid discrimination and ensure equitable treatment. It’s essential to prevent unjust consequences and ensure AI benefits all individuals and groups equally.
5. How can we mitigate bias in AI systems?
Bias can be mitigated through techniques like re-weighting data, re-sampling data, debiasing algorithms, and conducting regular audits and transparency measures.
6. What is the role of diverse and inclusive teams in ethical AI development?
Diverse and inclusive teams bring different perspectives and experiences, helping to identify and address biases and ethical issues in AI development.
7. Are there regulations and guidelines for ethical AI?
Yes, regulations like GDPR and guidelines from organizations like the IEEE and ACM provide ethical AI development and usage frameworks.
8. What is the future of ethical AI?
The future of ethical AI involves ongoing efforts to minimize bias, promote transparency, and ensure that AI technologies align with societal values. It will likely see increased regulations and a growing emphasis on responsible AI practices.
9. Can AI ever be completely bias-free?
Achieving complete bias-free AI is challenging, but we can significantly reduce biases and strive for Fairness through diligent efforts in data collection, preprocessing, and algorithm design.
10. How can individuals and organizations promote ethical AI in practice?
Individuals and organizations can promote ethical AI by prioritizing Fairness, transparency, and accountability, following ethical guidelines, and actively addressing bias in AI systems throughout their lifecycle.
Reference Sites:
Here are some reference websites related to your topic, “Ethical Considerations in AI: Bias and Fairness,” where you can find in-depth information, research papers, articles, and resources:
Website: AI Ethics Resources – Stanford
Website: IEEE AI Ethics
Website: Fairness and Machine Learning – Google
Website: AI Now Institute
Website: AI Ethics and Society – OpenAI
Website: Partnership on AI
Website: FAT/ML Conference
Website: AI and Ethics – The Hastings Center
Website: AI Ethics Toolkit – Markkula Center
Website: AI and Ethics Journal